To begin, a criticism
I picked up the Haskell Data Analysis Cookbook. The book presents examples of comparing data using Pearson Coefficient and using Cosine Similarity.
pearson xs ys = (n * sxy - sx * sy) / sqrt ((n * sxx - sx * sx) * (n * syy - sy * sy))
where
n = fromIntegral (length xs)
sx = sum xs
sy = sum ys
sxx = sum $ zipWith (*) xs ys
syy = sum $ zipWith (*) ys ys
sxy = sum $ zipWith (*) xs ys
cosine xs ys = dot d1 d2 / (len d1 * len d2)
where
dot a b = sum $ zipWith (*) a b
len a = sqrt $ dot a a
Although these code snippets are both calculating the ‘similarity’ between two vectors and actually, as we shall see, share a lot of structure, this is not at all apparent from a glance.
We can fix that however…
Definition of an Inner Product
An inner product is conceptually a way to see how long a vector is after projecting it along another (inside some space).
Formally, an inner product is a binary operator satisfying the following properties
Linearity

for 
We are saying that we can push sums inside on the left to being outside. We can also push out constant factors.
(Conjugate) Symmetry
or in the complex case, 
In the real case, we’re saying everything is symmetric – it doesn’t matter which way you do it. In the complex case we have to reflect things by taking the conjugate.
Positive Definiteness
with equality iff 
Here we’re saying projecting a vector onto itself always results in a positive length. Secondly, the only way we can end up with a result of zero is if the vector itself is of length 0.
From Inner Product to a notion of ‘length’
Intuitively a distance between two things must be
- positive or zero (a negative distance makes not too much sense), with a length of zero corresponding to the zero vector
- linear (if we scale the vector threefold, the length should also increase threefold)
Given that
we might be tempted to set
but then upon scaling
we get
– we’re not scaling linearly.
Instead defining
everything is good!
Similarity
Now, in the abstract, how similar are two vectors?
How about we first stop caring about how long they are, and want them just to point in the same direction. We can project one along the other and see how much it changes in length (shrinks).
Projecting is kind of like seeing what its component is in that direction – i.e. considering 2-dimensional vectors in the plane, projecting a vector onto a unit vector in the
direction will tell you the
component of that vector.
Let’s call two vectors
and
.
Firstly let’s scale them to be both of unit length, 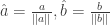
Now, project one onto the other (remember we’re not caring about order because of symmetry).

Using linearity we can pull some stuff out (and also assuming everything’s happily a real vector – not caring about taking conjugates)…

Making Everything Concrete
Euclidean Inner Product
The dot product we know and love.

Plugging that into the similarity formula, we end up with the cosine similarity we started with!
Covariance Inner Product
The covariance between two vectors is defined as
where we’re abusing the notion of expectation somewhat. This in fact works if X and Y are arbitrary L2 random variables… but for the very concrete case of finite vectors we could consider
.
We’ve said in our space, to project a first vector onto a second we see how covariant the first is with the second – if they move together or not.
Plugging this inner product into the similarity formula, we instead get the pearson coefficient!
In fact, given
, in this space we have
,
i.e.
.
Improving the code
Now that we know this structure exists, I posit the following as being better
similarity ip xs ys = (ip xs ys) / ( (len xs) * (len ys) )
where len xs = sqrt(ip xs xs)
-- the inner products
dot xs ys = sum $ zipWith (*) xs ys
covariance xs ys = exy - (ex * ey)
where e xs = sum xs / (fromIntegral $ length xs)
exy = e $ zipWith (*) xs ys
ex = e xs
ey = e ys
-- the similarity functions
cosineSimilarity = similarity dot
pearsonSimilarity = similarity covariance
Things I’m yet to think about
…though maybe the answers are apparent.
We have a whole load of inner products available to us. What does it mean to use those inner products?
E.g.
on
– the inner product producing the Fourier transform. I’m not the resulting similarity is anything particularly special though…